Best Practises for Data Annotation in Deep Learning Models
- D
- Sep 3, 2024
- 3 min read
Master the art of data annotation to enhance the accuracy and reliability of your deep learning models.
Understanding the Role of Data Annotation in Deep Learning
In the realm of deep learning, data annotation serves as the foundational cornerstone. Without meticulously labeled data, deep learning models would struggle to learn and make accurate predictions. Dataset annotation involves the process of labeling data to teach the model what it needs to recognise, whether it’s identifying objects in images, transcribing speech, or categorising text.
The importance of data annotation cannot be overstated. It ensures that the input data is both accurate and reflective of real-world conditions, which is critical for training models that produce reliable and actionable outcomes. By providing high-quality annotations, we can significantly improve the performance and accuracy of deep learning
Key Principles for Effective Data Annotation
Effective annotation hinges on several key principles. First and foremost, clarity and consistency in labelling are paramount. Data Alike's annotators must follow a well-defined set of guidelines to ensure that each data point is labeled in a consistent manner. This helps in maintaining uniformity and reduces the introduction of bias.

Another crucial principle is the use of domain experts. Leveraging individuals with specific knowledge about the data being annotated can significantly enhance the quality of the annotations. Additionally, iterative refinement, where initial annotations are reviewed and refined, ensures that any errors or inconsistencies are addressed promptly.
Tools and Technologies for Efficient Data Annotation
There are numerous tools and technologies available that facilitate efficient dataset annotation. These tools range from simple labelling interfaces to sophisticated platforms
that incorporate machine learning to assist in the annotation process. Popular tools include Labelbox, Amazon SageMaker Ground Truth, and VGG Image Annotator, each offering unique features tailored to different annotation needs. Our annotators are able to adopt whichever tool your company prefers.
In addition to these tools, technologies such as automated annotation and active learning can significantly speed up the annotation process. Automated annotation uses pre-trained models to label data, which can then be reviewed and corrected by our human annotators. Active learning, on the other hand, involves the model actively querying the annotator for the most informative samples, thereby optimizing the annotation workflow.
Common Challenges and How to Overcome Them
Data annotation comes with its set of challenges. One of the most common issues is the introduction of annotation bias, where personal biases of annotators influence the labelling process. Data Alike mitigates this by establishing and following the clear and comprehensive guidelines set by our clients. We also conduct regular training sessions for our annotators.
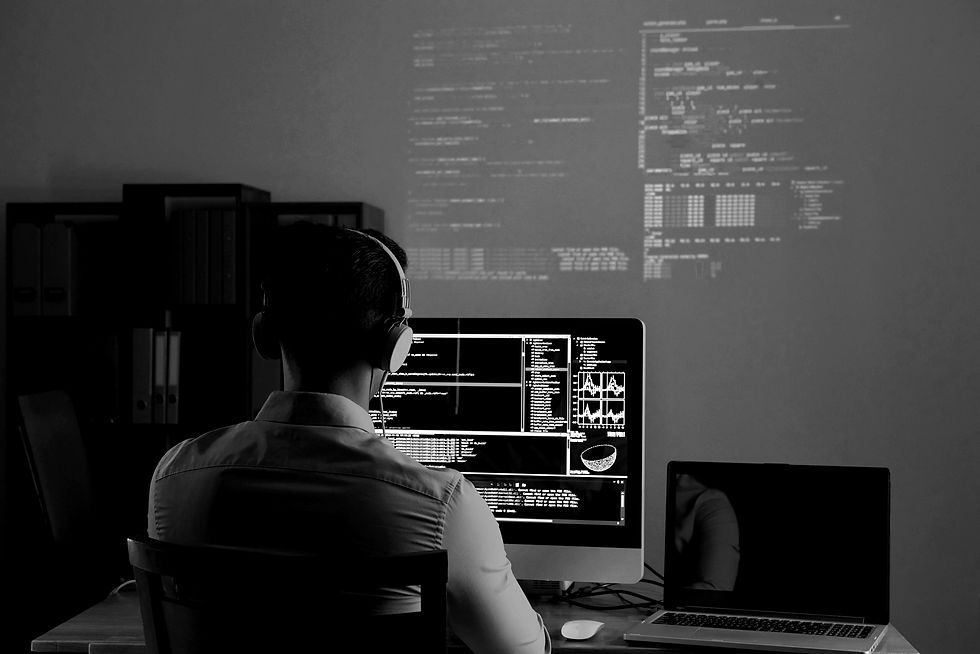
Another challenge is dealing with large volumes of data, which can be both time-consuming and resource-intensive. Strategies such as outsourcing, where a company such as Data Alike provides multiple annotators to contribute to the labelling process is a good option. Utilising automation tools can also help manage the workload effectively. Additionally, we like to implement a quality control mechanism, such as cross-validation by multiple annotators and regular audits, to ensure the reliability and accuracy of our annotations.
Future Trends in Data Annotation for Deep Learning
The future of dataset annotation is poised for significant advancements, driven by emerging technologies and methodologies. One notable trend is the increased use of artificial intelligence to aid the annotation process. AI-powered tools can perform initial annotations, which can then be refined by our human annotators, drastically reducing the time and effort required.
Another trend is the integration of more sophisticated active learning techniques, where the model intelligently selects the most informative samples for annotation. This not only improves the efficiency of the annotation process but also enhances the model's learning capabilities. Additionally, the development of better annotation standards and practices will likely continue to evolve, ensuring higher quality and more consistent annotations across various domains.
Enhance your machine learning projects with our precise data annotation services. Contact Data Alike, for standout annotation services.